Data Transformation
Data transformation is a crucial step in preparing your data for meaningful analysis. We specialize in converting raw data into a structured format that is easy to analyze and interpret. From normalization and scaling to encoding categorical variables, our data transformation services ensure that your dataset is optimized for accurate statistical modeling. Let us help you unlock the full potential of your data for actionable insights and better decision-making.
Get in Touch with Our Statistics Experts
Fill out the form below, and our team will get back to you shortly.
Unlock the Power of Data Transformation for Precise Analysis
Data transformation is crucial for optimizing datasets, ensuring consistency, and enabling effective analysis, leading to deeper insights and better decision-making.
Improved Data Consistency
Data transformation standardizes and harmonizes data, ensuring consistency across datasets.
Enhanced Data Quality
Transformed data is cleaner, more accurate, and ready for analysis, improving the reliability of results.
Simplified Data Analysis
Transforming data into usable formats makes analysis more straightforward, reducing complexity.
Improved Model Performance
Correcting and normalizing data enhances the performance of predictive models, leading to more accurate forecasts.
Outlier Detection and Removal
Data transformation helps identify and manage outliers, ensuring they don’t distort the analysis.
Facilitates Better Decision-Making
Clean, transformed data enables clearer insights and supports better strategic decisions.
Order Now to Get Started
Documents Required
Data transformation is a critical step in preparing raw data for analysis, ensuring it is structured and formatted according to the requirements of your study or model. To effectively transform your data, we require specific documents that provide a clear understanding of the data’s structure, desired output, and any transformation rules.
Raw Data Files (Excel, CSV, or other formats)
Data Transformation Guidelines or Specifications
Data Dictionary or Variable Definitions
Desired Data Format and Structure
Data Collection Methods Documentation
Sample Data for Review and Testing
Previous Data Transformation Reports (if applicable)
Business Rules or Constraints for Transformation
Data Validation Requirements
Permissions for Data Access or Modification
Timeline Process
Assess Data Structure
Examine the dataset to understand its format, variables, and relationships, ensuring it’s suitable for the intended analysis.
Define Transformation Goals
Determine the specific transformations needed, such as normalization, encoding, or aggregation, based on the analysis requirements.
Apply Transformations
Implement the required data transformations, such as converting variables, scaling values, or creating new derived features.
Verify Transformed Data
Check the transformed dataset to ensure all changes were applied correctly and that the data is now in the desired format for analysis.
Document the Process
Record the transformation steps taken, including the rationale behind each, to ensure transparency and reproducibility of the process.
Find the Perfect Fit for Your Budget
Choose from our range of flexible pricing options that cater to your specific needs.
₹5,999
Basic Plan
A brief description goes here
₹12,999
standard Plan
A brief description goes here
₹25,999
premium Plan
A brief description goes here
₹49,999
Enterprise Plan
A brief description goes here
Simplifying Everyday Life
Frequently Asked Questions
Find answers to commonly asked questions about our services.
What is data transformation in statistics
Data transformation is the process of converting data from its original format or structure into a format suitable for analysis, often to meet statistical assumptions or improve model performance.
Why is data transformation important in statistical analysis
Data transformation helps to make data more suitable for analysis, improve normality, stabilize variance, and enhance the effectiveness of statistical models, especially when data doesn’t meet assumptions.
What are the common types of data transformations
Common transformations include logarithmic transformations, square root transformations, inverse transformations, and Z-score standardization, among others, depending on the nature of the data.
When should I apply data transformation
Data transformation is typically applied when data is skewed, contains outliers, violates assumptions of normality, or when variables need to be brought to a comparable scale for modeling.
What is normalization in data transformation
Normalization is the process of adjusting the values in a dataset to a common scale, typically between 0 and 1, to ensure that variables with different units or scales can be compared.
What is standardization, and how does it differ from normalization
Standardization (or Z-score transformation) involves subtracting the mean and dividing by the standard deviation to make the data have a mean of 0 and a standard deviation of 1. Unlike normalization, it doesn’t constrain values within a fixed range.
How do I apply a logarithmic transformation to my data
A logarithmic transformation is applied by taking the natural logarithm (log) or base 10 log of each data value, often used to correct for skewness or reduce the impact of outliers in highly skewed data.
What are the benefits of using data transformation in statistical modeling
Data transformation can improve the accuracy and efficiency of statistical models by meeting assumptions like normality, linearity, and homoscedasticity, which are essential for valid results in techniques such as regression analysis.
Submit Your Request
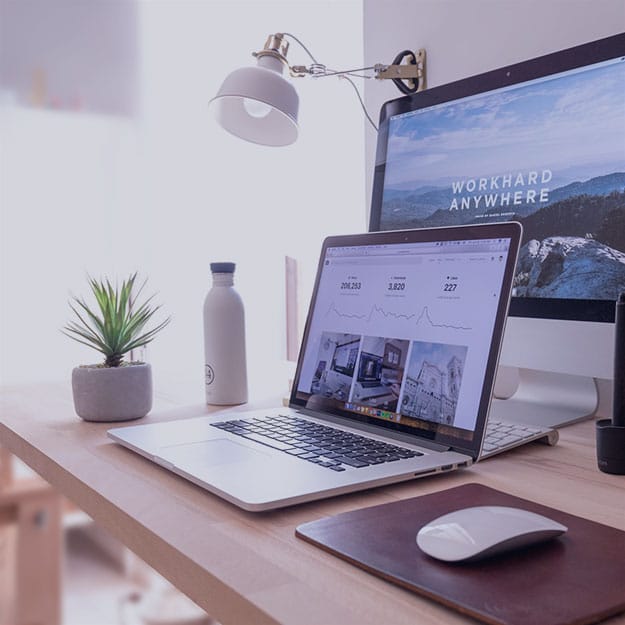