Get in Touch with Our Statistics Experts
Fill out the form below, and our team will get back to you shortly.
Timeline Process
Data Collection
Gather historical sales data, seasonal trends, and external factors like economic conditions or promotional activities that could influence demand.
Data Cleaning and Preparation
Preprocess the collected data by handling missing values, correcting inconsistencies, and ensuring the dataset is ready for analysis.
Trend and Seasonality Analysis
Identify trends, seasonal patterns, and cyclic behavior in the data to understand demand fluctuations and forecast future behavior more accurately.
Model Selection
Choose an appropriate forecasting model, such as time series analysis, ARIMA, or exponential smoothing, based on the nature of the demand data.
Model Development
Develop and fit the forecasting model using historical data, applying statistical methods to predict future demand based on identified patterns and trends.
Model Validation
Validate the accuracy of the model by comparing predicted demand with actual values, using performance metrics like Mean Absolute Error (MAE) or Root Mean Squared Error (RMSE).
Forecasting and Reporting
Generate demand forecasts for the desired time period and prepare a report with actionable insights, highlighting expected trends and supporting decision-making for inventory, staffing, and production planning.
Submit Your Request
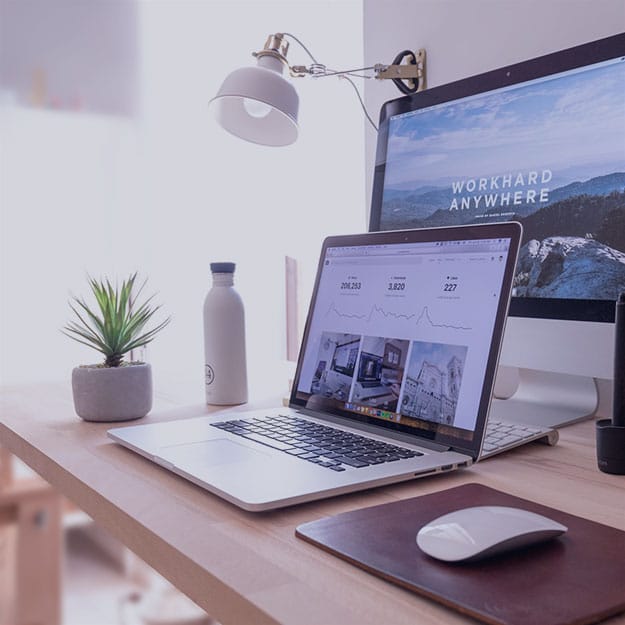