Get in Touch with Our Statistics Experts
Fill out the form below, and our team will get back to you shortly.
Timeline Process
Data Collection and Integration
Collect customer data from various sources, including transactional history, customer interactions, and behavioral patterns to build a comprehensive dataset.
Data Preprocessing
Prepare the data by handling missing values, correcting errors, and transforming data into a usable format for analysis.
Feature Engineering
Identify and create relevant features, such as customer engagement metrics and usage patterns, that are strong predictors of churn.
Exploratory Data Analysis (EDA)
Analyze the dataset to identify trends, correlations, and patterns that may indicate early signs of customer churn.
Model Selection and Training
Choose suitable statistical or machine learning models (like logistic regression or decision trees) to predict churn and train them on the historical data.
Model Validation and Testing
Evaluate the model’s accuracy, precision, and recall using testing data to ensure its predictive reliability.
Risk Segmentation
Segment customers into different risk groups based on their likelihood of churning, helping prioritize retention efforts.
Implementation and Monitoring
Deploy the churn prediction model in real-time systems and continuously monitor its performance to make improvements and updates as needed.
Submit Your Request
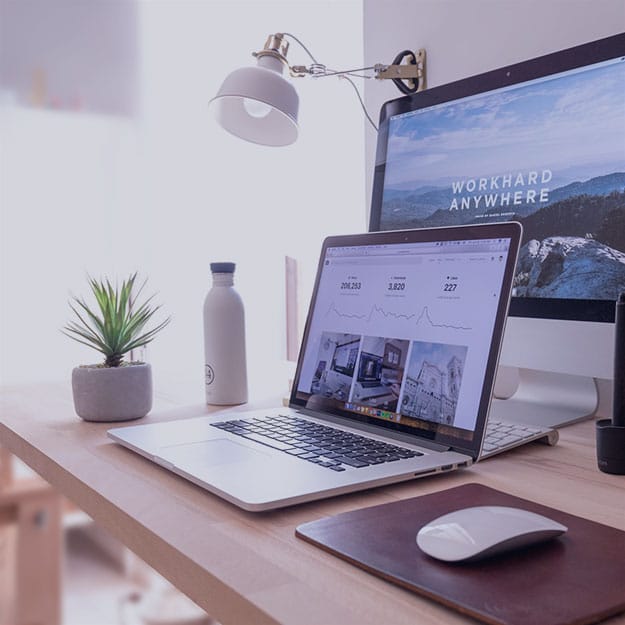